Life sciences have never been more digital. To learn more about life processes, biologists are collecting massive quantities of data that computer scientists analyze by means of sophisticated computational models that they develop. Over the past few years, Dr. Ori Avinoam of the Biomolecular Sciences Department at the Weizmann Institute of Science has been grappling with one unresolved biological conundrum: How do stem cells generate new muscle fibers? In his search for an answer, Avinoam turned to his friend Dr. Assaf Zaritsky from the Software and Information Systems Engineering Department at Ben-Gurion University of the Negev, and together they started developing a machine learning model capable of tracking this complex biological process. As the researchers reported recently in Molecular Systems Biology, their model could attach numerical scores to each cell in the course of its unique maturation – and this allowed them to define a novel regulatory checkpoint in this process.
The stem cells from which muscle tissue develops are created in the embryo, but a few of them are still present in adult muscles. These cells are dormant most of the time, but during growth, strenuous physical activity or injury they leap into action. At the first stage, the stem cells divide in order to increase their numbers. They then stop dividing and undergo what is known as differentiation – a part of the maturation process in which cells specialize in performing a unique function and acquire traits necessary to fulfill it. In the case of muscle tissue, the differentiating stem cells become elongated, begin to synthesize the protein fibers that give muscles their characteristic ability to contract and then migrate to wherever the tissue is regenerating. Once they arrive at their destination, they fuse together to form one long cell, known as muscle fiber. A collection of these cells is what makes up the entire muscle. Until now, however, scientists have had difficulty understanding how stem cells progress along this path of specialization and what causes them to move from one stage to another.
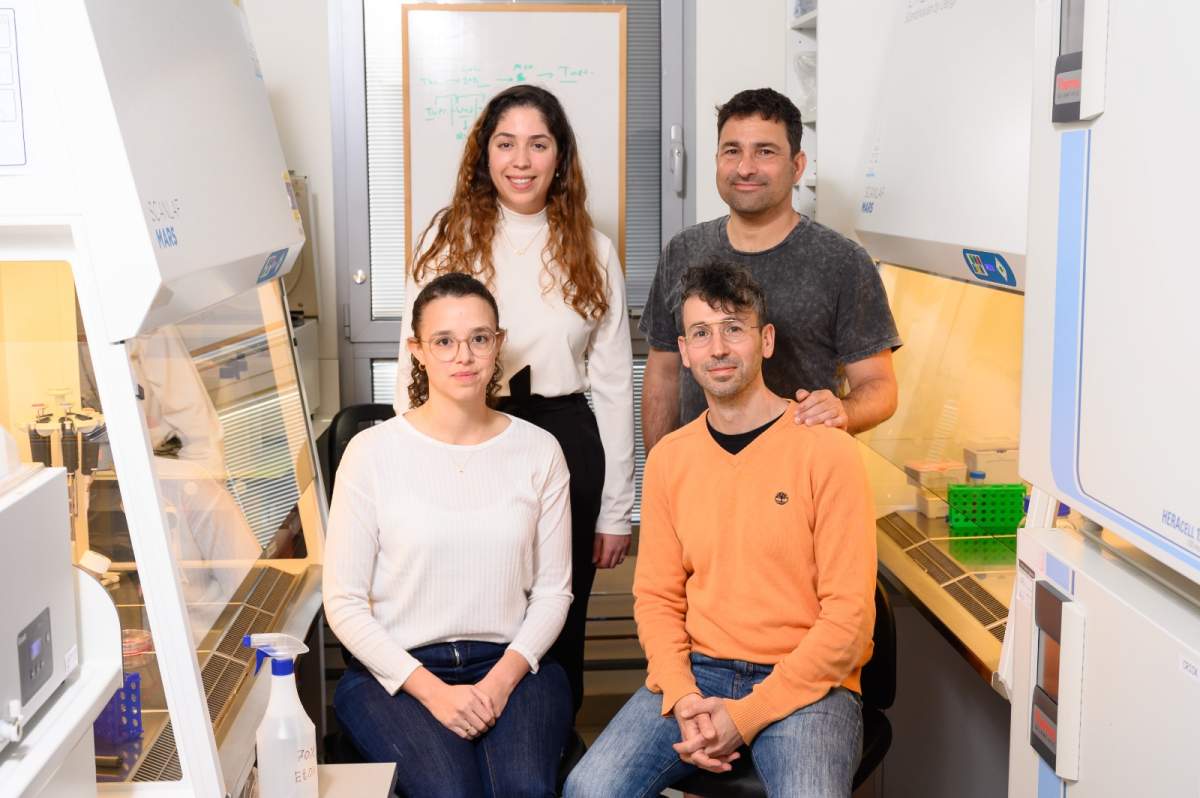
Seeking to address these questions, Giulia Zarfati and Adi Hazak from Avinoam’s lab documented in real time how muscle fibers develop from stem cells isolated from mice. They decided to focus on two changes: the movement of the cells and the manufacture of protein fibers inside them, which is essential for generating an adult muscle capable of contracting. To follow the movement of these cells, the researchers fluorescently labeled their nuclei and one of the protein components, called actin, that is essential for making fibers. Throughout a day-long differentiation process, the researchers created numerous videos documenting, down to the level of a single cell, the stages by which hundreds of stem cells become adult muscle cells and fuse into a new fiber.
“The two research groups had to learn each other’s language. Assaf’s team learned what a differentiated muscle cell is and my team had to study the basics of machine learning”
Having collected abundant biological data, the scientists teamed up with research student Amit Shakarchy from Zaritsky’s lab to build a model that would accurately represent this dynamic process. “The two research groups had to learn each other’s language,” Avinoam explains. “Assaf’s team learned what a differentiated muscle cell is and how we know when it has fused with other cells to form muscle fiber. My team had to study the basics of machine learning and how to analyze data collected from a sequence of observations at different times. Then we had to work out together how to translate the biological process into a computational model capable of following its progress.”
Building a computerized model that can monitor a dynamic biological process is a huge challenge. “First, we had to decide how to define the point in time at which a cell was differentiated,” Zaritsky explains. “After that, we had to choose whether and how to use this temporal information. We decided to incorporate it while training a supervised model that follows the movement of the cells and the intensity of the fluorescent light emitted by the actin fibers they contain. The model also examined derivatives of these data, such as changes in the cells’ motion speed and how the actin fibers’ structure changes over time.”
The video shows the unique path followed by several individual muscle cells on their way to fusing and becoming a new muscle fiber
The researchers discovered that, as the differentiation process progressed, the cells’ motility decreased, whereas the strength of the signal from their actin fibers increased. The machine learning model, trained to distinguish between stem cells and adult muscle cells, created a real-time quantitative index that gives a numerical score to each individual cell, based on how far along it has progressed in its differentiation. When the model was tested on experiments for which it had not been trained, the researchers found that most of the stem cells gradually scored higher during the differentiation process, reaching the highest mark when the process was completed. “The model showed us that differentiation is a gradual and decentralized process, so that the cells do not progress together in stages – rather, they follow different patterns of progress,” Avinoam says. “That was an unexpected finding, since we assumed that the cells would display collective behavior.”
“The ability to continuously follow cells transitioning in real time could help us in the future to monitor the progress of diseases in an unprecedented way. Today, for example, we examine cancerous growths by taking a biopsy, a sampling that is frozen in time and does not provide us with ongoing information about a dynamic biological process,” Zaritsky adds.
Stop before you fuse
Although the model suggested that different cells complete their maturation process at different times, it also found that from the moment of completion on, there is a consistent period of around three hours before they fuse together and become muscle fiber. This led the researchers to postulate that at a certain checkpoint, each cell makes sure that it has indeed finished differentiating, and only then sets the fusion process in motion.
Past studies had suggested that an enzyme called p38 regulates muscle development, but its precise role was unknown. To test whether the enzyme was the crucial component of the checkpoint step, the researchers inhibited its activity and found that, indeed, the cells got stuck: They did not fuse into a new muscle fiber.
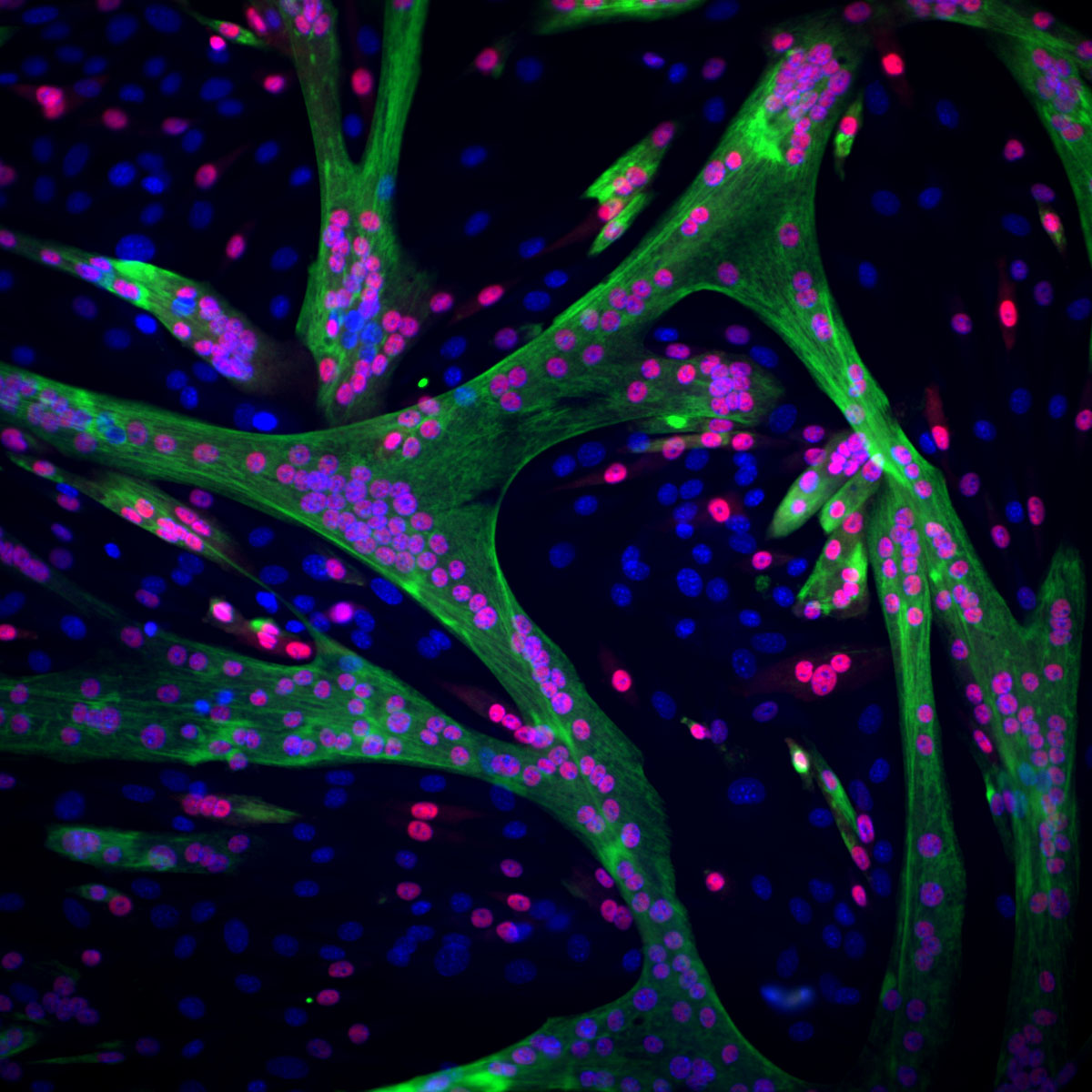
When the researchers ran the computational model, they saw that the cells in which the enzyme had been blocked were given a numerical score that continued to rise. In other words, even in the absence of the enzyme, they successfully completed their differentiation process but did not continue to the fusion stage. The researchers concluded that the checkpoint comes at the end of the differentiation process but before the fusion stage. But why did the cells become stuck at this step in the absence of the enzyme?
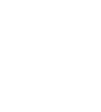
The human back thigh muscle, biceps femoris, contains more than 37.5 million cell nuclei. It is made up of more than 250,000 muscle fibers, each of which contains an average of 150 cell nuclei after the differentiation and fusion processes.
The model suggested one possible explanation, showing that when the enzyme’s activity was inhibited, actin fibers became organized in a different manner during differentiation. When the researchers measured the level of protein expressed in inhibited cells, the findings confirmed the model’s prediction: The cells expressed a high level of the proteins that are responsible for organizing the actin fibers in the cytoskeleton – an important stage in the differentiation process and in readying the cells for fusion. At the same time, the cells had lower levels of the proteins that are needed for fusion, those that help create adult muscle fibers and allow the muscles to contract. “The cells get stuck in a stage of ‘ready-to-fuse,’” says Avinoam. “So, when the enzyme becomes active again, they can resume the fusion process. In fact, we believe this is the central checkpoint at which the muscle ensures that its cells have completed their preparation for fusing into a new muscle fiber. Beyond shedding new light on muscle development, this discovery shows that computerized models are capable of identifying important checkpoints in dynamic biological processes.”
Recent Comments